Forecasting demand, and then balancing it with supply chain capabilities, has never been straightforward – there was no Golden Age when the customer’s economic order quantity bore any resemblance to the manufacturer’s economic batch quantity. And, says Sam Tulip, demand management is becoming more complex.
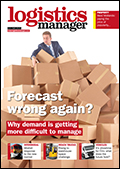
Retailers and manufacturers are ever less sure how demand for even their core lines will develop, while the ‘long tail’ of products with slow or intermittent demand grows inexorably.
There are many drivers for this. There is a proliferation of products and of sources – customers are no longer tied to whatever their local manufacturer or retailer chooses to offer (‘Any colour so long as it’s black’ is no longer a strategy: cars are built to individual, highly customised, order). Many products have much shorter life cycles than hitherto and many more are now fashion choices rather than basic needs – it used to be you joined a waiting list for a telephone: now it is a disposable lifestyle accessory. Business customers are taking a more granular approach to forecasting, and replenishing more often but in smaller quantities, while increasingly businesses are selling direct to end users in small quantities rather than manufacturing to bulk demand from dealers.
Meanwhile both B2B and B2C customers have ever higher service expectations.
“Demand Management is where it all starts”, says Hank Canitz, product marketing director at Logility. “It provides the information for all the rest of the planning – inventory, deployment strategy, manufacturing, purchasing, even manpower”. But there are a lot of challenges. Eric Carter, Solutions Architect at Indigo Software, estimates that 80 per cent of companies get their forecasts wrong 80 per cent of the time.
Forecasting, says Canitz, is still mostly based on historic sales “but this is the wrong metric. Order history is better – when and where the customer wanted it, not when you shipped and where from. If you don’t clean the history up, things soon get misaligned”.
Companies struggle with creating forecasts at the appropriate level – should it be at sku level, or at a higher aggregation. “It can be trial and error to determine the best level to forecast at, and how to bring the forecast down to customer level”. Choosing the appropriate forecasting algorithm is also problematic: lots of companies use one method across all their skus and forecast: the model may fit 60 per cent of the business but be suboptimal for, say, slow moving lines, intermittent demand, or seasonal peaks.
Every product has a lifecycle, and forecasting for a new product introduction is very different from an end of cycle product. Then ‘events’ including promotions must be added.
BOGOF bother
Promotions deserve special notice. Your own promotion should create higher demand, but you may benefit also from a competitor’s promotion lifting the entire sector. “It is super-important”, says Hans-Georg Kaltenbrunner, vice president EMEA industry strategy at JDA Software, “to factor in all the promotions” and similar business intelligence. (He also points out that comparable activity is becoming more important in B2B, as manufacturers negotiate rebates with private brands, or discounts for flexible scheduling).
Andrew Blatherwick, chairman of Relex, adds that other externalities include “extreme weather which is beginning to drive more complexity, and even Brexit”. Technology may help. FuturMaster is working on new tools using machine learning and artificial intelligence for promotions forecasting. “Instead of focusing on volumes, these tools should dictate what will be the most profitable products, for how long, and at what particular time of year”, according to FuturMaster’s Stephanie Duvault-Alexandre.
Another feature of promotional demand noted by Blatherwick is that, not infrequently, a promotion is planned without reference to the ability of individual stores to accommodate it adequately, or without cannibalising other sales. This reinforces the message that demand management cannot just exist in high level aggregates.
Kaltenbrunner suggests the need for multiple flavours in the forecast recipe: “Obviously, take a collaborative approach – ask the customer! Then you can use statistical forecasting and machine calculated algorithms for seasonality, new product impact on existing products, how much you can expect to steal from the competition. Include historical data. And there will be manual overrides by field sales and market intelligence teams. In theory these should all align towards a consensus figure that will drive the supply chain. But things can go wrong”.
Demand forecasting cannot be totally automated but it is important to know if and when human overrides are improving things. Kaltenbrunner cites a white goods manufacturer’s analysis – “in two thirds of cases input from field sales did not improve the forecast, which would have been better if it had simply repeated the previous month’s figures!” Canitz agrees. “If you have a finely tuned statistical forecast, 9 times out of 10 letting the sales force kick it around will make it worse. They want to satisfy customers without a care for excess inventory. As a demand planner you have to understand that, and insist they only add information that is significant”.
Kaltenbrunner suggests using methods like segmentation – “focus on clusters where it matters; leave the rest to automation, or even do nothing”. Clusters, he says, are fairly well understood, but not often applied in this context”.
Technology to the rescue
Technology can help to a point, says Canitz. It can select and test for the right algorithm and forecast period. Filters can identify spikes in the history (of known or unknown causes) and adjust the forecast. And they can flag the items with the highest forecast errors in relation to profit or revenue (as Kaltenbrunner points out, the questions are as much about managing revenue as managing demand, and the two are inseparable). Systems can also model demand for a new product by looking for previous products with similar characteristics. With tens or even hundreds of thousands of skus, this sort of analysis is impossible manually.
“There is a plethora of forecasting tools available”, comments Nitin Aggarwal, vice president Data Analytics at The Smart Cube. “Most ERP systems such as SAP and Oracle, and supply chain management software such as JDA or Llamasoft have in-built demand forecasting. Then there are specialist forecasting tools – Forecast Pro, Autobox, GMDH Shell etc. Lastly, there are open source packages now emerging that will democratise demand forecasting algorithms: recently, Facebook released an open source algorithm called Prophet for forecasting time series data”. Aggarwal adds that machine learning is now widely used, and that Artificial Neural Networks are beginning to make an impact.
Kaltenbrunner suggests that Cloud technology can bring manufacturers and retailers together in a more collaborative approach based on looking at the flow. A flowcasting engine can be pre-populated with planning logic and rules reflecting everyone’s plans, objectives and requirements. “You can add in, for example, promotions, and feed the results to the sales force. Everyone gains visibility of how orders would flow through both ends of the supply chain and down to shop level”.
Steep learning curve for e-commerce
On-line retailers are particularly exposed to rapidly changing flows and demand patterns, resulting, says Andrew Blatherwick, in “piles of inventory”, moving around or gathering dust. An online division or brand rapidly becomes a silo, inefficiently holding duplicate stock. Multiple stock holdings lead inexorably to the extremes of markdowns and stock-outs. Demand should be reconciled through the rules of the system. “Use the rules to run the business and change priorities. If an end of cycle product is down to a week’s stock, offer it online only by changing the rules, not by moving the boxes”.
Jonathan Bellwood is chief executive of Peoplevox, which specialises in SaaS solutions for e-commerce. “One of the biggest challenges for our clients is their very big sku base, typically 100,000+. The money is in the stock, and they have a very long tail tying money up because they haven’t planned for demand, and they don’t know what they should be buying”.
Many companies forecast by looking at what has sold over a comparable period, or during a similar event and stick their finger in the air. “What they fail to consider is: how many days were the goods actually in stock? Perhaps they sold out in the first two days so didn’t meet the demand that was there. They need to know the rate of sales (hourly, daily, weekly) when the items were in stock.
“Then,” Bellwood continues, “they need to go back to their web site. Ask what was the conversion rate, and combine that with when the goods were in stock. The items to buy are those with a high conversion rate and a high availability.
“It sounds like a statement of the blindingly obvious, and it is. The tools are there: take the web page, some Google Analytics, your stock in and out, and bingo!”
Bellwood maintains that 10-30 per cent of e-commerce stock is “totally wrong – bin it, discount it. It’s gathering dust in the warehouse, and no, you won’t sell it next year. But some of our clients buy stock to have something to sell, a picture to put up on the website, even if they don’t think it will sell”.
Bellwood continues “e-commerce still has growth and money is cheap – as long as the sales growth is there, people forget about the real costs. They invest to save a small percentage of their IT transaction costs and forget about the money sitting on shelves because they didn’t understand demand”.
Going spare
Spares, repairs and aftermarkets have particular pain points – the ‘long tail’ with slow, intermittent, inherently unpredictable demand. Gill Devine, vice president enterprise sales western Europe for Syncron, understands the conflict between reducing levels of near-obsolete stock, and of increasing customer service when an asset is down. Additionally, in the aftermarket, “there are a lot more locations and, as with dealer networks, they aren’t necessarily yours. Your business reputation is controlled by others. How do you maintain visibility across thousands of locations so that you can source from within the supply chain rather than from new manufacturing?”
A screw may be a commodity item; a replacement turbine could take years to manufacture. The aftermarket supplier needs to know where everything is and what the lead times from those locations are. Additionally, many firms handle repairables, which may be returned to their owner, or entered into stock – firms need to know where they are and in what condition. And of course you don’t want to hold parts in a country where equipment is no longer used.
Devine says “We start with new clients by looking at the data layer – master data cleansing. It is common for example for parts to be used in different equipment with different part numbers: it is hard to see what demand for that part is or how many you have got”. Carter of Indigo agrees: “Without a high level of confidence in the accuracy of stock information, it’s all wasted effort”.
“Forecasting is only one part: we have to relate the forecast to expected and intended service levels. Segment and optimise service levels: fast moving low cost items require a different approach from slow moving, expensive, but vital parts. We simulate service levels to see the impact on cost, trying to maximise service at minimum cost of inventory”.
New products, says Devine, present a particular challenge, there being no historical data. “Our inventory optimisation tools can look at historicals for similar products and see what the patterns of demand have been. If you launch a new vehicle, you should at least be able to fulfil the predictable demand. And parts aren’t necessarily unique to you – people will go elsewhere and not come back. Parts that can be got elsewhere must be available”.
Data sharing is key, says Devine. “We can do virtual planning in virtual warehousing. In Australia, with huge distances, we have to be able to let networks see what’s available, where and with what lead time. We can let the dealer network see the whole picture, and allow movement of parts between dealers. This can be as open as an OEM wants it to be” [even to external ‘competitors’].
Demanding shelves, needy fridges
Of RFID, Andrew Blatherwick predicts “In a couple of years a tag will be a cent: at that price it is applicable to food and the world will change. The supply chain is absolutely driven by accurate information. RFID gives you, quickly and accurately, how much stock you’ve got and where it is, in real time, all the time” and therefore, of course, what replenishment you need.
Internet of Things technologies mean that smart shelves, smart fridges, even smart waste bins could generate orders for direct replenishment to store or home. Self monitoring spares and aftermarket applications are also obvious. Syncron’s Devine says “IoT is having an impact on industries which are selling ‘uptime’ contracts rather than just parts. We are beginning to see monitoring of machines in the field, and predictive analytics on vehicles, feeding back in to the demand signal”. Blatherwick says “Ultimately this is likely to happen more widely: it could make a huge difference, and it could happen very quickly. Smaller retailers can afford the same sort of solution that major retailers have enjoyed and will become more rather than less competitive. It’s not quite at Mom and Pop store level yet, but it will be”.
Some cautionary notes. Logility’s Hank Canitz points out that “demand management provides the S in Sales & Operations Planning, which is pretty important. But it’s unconstrained planning, consensus demand planning, and a lot of companies are only doing the S. They have to apply the constraints of people, production, transport and so on to come up with a plan which says ‘This is what we can make, this is what we can sell, and this is what the profit is. That’s what’s supposed to come out of the meeting.
“And there is talk of running S&OP effectively in real time powered by the latest demand signals. But they are missing the point. The forecast and the plan has to look far enough into the future to give everyone a chance to make the changes needed to make the plan work, make it meet the demand”.
And whatever the technology, demand management is in the end about matching human needs and capabilities. Mark Feather, regional marketing manager EMEA for the DynaSys division of QAD, notes “Demand planners need to have a skill set that combines the science, the statistical forecast, with the art, the collaborative input. They must be super-users of the planning tools as well as being able to build relationships with the commercial team and convert their insight into actionable forecasts”.